Using Analytics To Detect Legal Risk
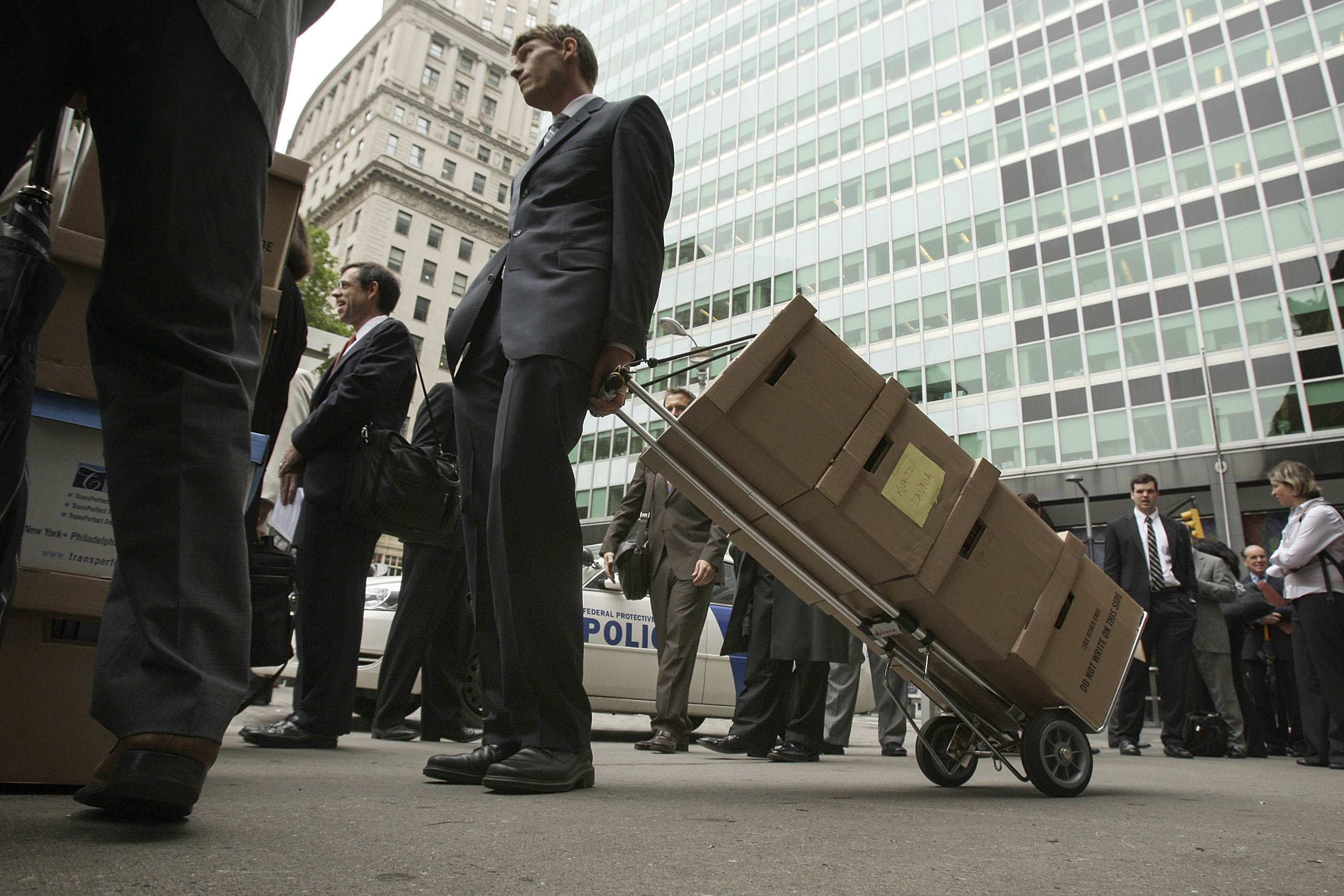
Lawyers with boxes of documents line up outside the United States Bankruptcy Court for the Southern District of New York. Technological advances will soon increase the speed and efficiency of legal tasks, such as analyzing masses of documents.
Photo: Spencer Platt/Getty Images
If the job of a lawyer were reduced to its essential tasks, analyzing text and making predictions would be high on the list: What contract terms present litigation risk? How might a judge rule in a lawsuit, given the precedent and the facts of a case? What internal policies pose the greatest regulatory and compliance risk for a company?
Historically, lawyers drew on their training and judgment, learned in law school and then honed by practice, to perform these tasks. However, today lawyers are increasingly relying on the tools of data science as well.
From analyzing contracts to tracking litigation metrics, advances in artificial intelligence, machine learning, natural language processing, programming, and high-performance computing are transforming legal services and revolutionizing the practice of law.
These new tools perform two main functions: increasing the speed and efficiency of legal tasks that previously required a large investment of labor hours and enabling the discovery of new insights that were previously buried in masses of documents or overlooked by manual analyses.
According to The New York Times, Forbes, and other outlets, “the implications are dizzying” regarding this application of data science to the practice of law. It is also big business. A recent study values the legal analytics market at $451 million in 2017 and projects that it will grow to $1.85 billion over the next five years.
Predicting Employee Complaints
An example illustrates legal analytics’ disruptive potential. Suppose that a company wants to get better at predicting which employee complaints lead to lawsuits. Historically, the company might assign a team of analysts and lawyers to comb through complaint records, personnel files, and court documents, searching for some set of variables thought to be predictors of litigation risk and manually testing those variables’ correlation with lawsuits filed. This painstaking process could take months and require an army of people to read and hand-process masses of text.
Treating this task as a data science problem dramatically improves speed and efficiency.
We are nowhere near robots taking over the practice of law. However, today’s lawyers and their clients can benefit from advances in analytics.
Text Mining
Text mining enables the extraction of key information from documents with great accuracy and in very large numbers. Machine learning allows an algorithm to “learn” which characteristics of an employee complaint are more and less predictive of litigation—testing all possible combinations to uncover patterns that the analysts and lawyers may not have thought to consider.
In a recent contest in the United Kingdom, for example, 100 lawyers from top London firms were pitted against an artificial intelligence tool to predict the outcome of hundreds of simple financial disputes, given a set of facts about each case. The robot won by a wide margin, predicting 86.6 percent of cases correctly, while the humans correctly predicted only 66.3 percent. The tool was “learning” something about the disputes that the humans were missing, beating lawyers at their own prediction game.
Not Mimicking the Human Touch
Here, the production of knowledge does not depend on the number of people available to throw at a task or on the constraints of pre-existing ideas about which information is relevant. Instead, insight flows directly from the subject matter itself. The analysis not only becomes quicker, but better.
As Andy Abbott, co-founder and CTO of Heretik, a legal tech software company, has commented, “We’ll see many attempts to mimic and simply automate existing [manual] processes. … However, those processes were developed and perfected with the tools at hand. As we introduce new tools and techniques, facilitated by machine learning, processes will evolve. Maturity will have reached the industry when we can look at a process and see no resemblance to how it was done it the past.”
Of course, not all legal questions and problems are neatly reducible to a set of variables, and human behavior does not always follow detectible patterns. Moreover, as observers such as Cathy O’Neil and Virginia Eubanks have pointed out, data often contain bias and inaccuracies, meaning that even the most sophisticated computer code, when fed garbage, can produce only garbage in return.
Robots Make Mistakes, Too
And perhaps more concerning, if we delegate too much of our decision-making to algorithms, which operate only on historical data, then we are destined to repeat our historical patterns and mistakes. In the end, a robot lawyer is a poor substitute for a human lawyer that has a human’s ability to exercise judgment and critically assess the patterns that data reveal.
We are nowhere near robots taking over the practice of law. However, today’s lawyers and the clients they represent can benefit from advances in analytics to increase speed and efficiency and detect patterns buried in data. These new insights become an additional resource that lawyers can deploy—alongside their own judgment and experience—in spotting, managing, and mitigating legal risk.