Why New York City Is Cracking Down on AI in Hiring
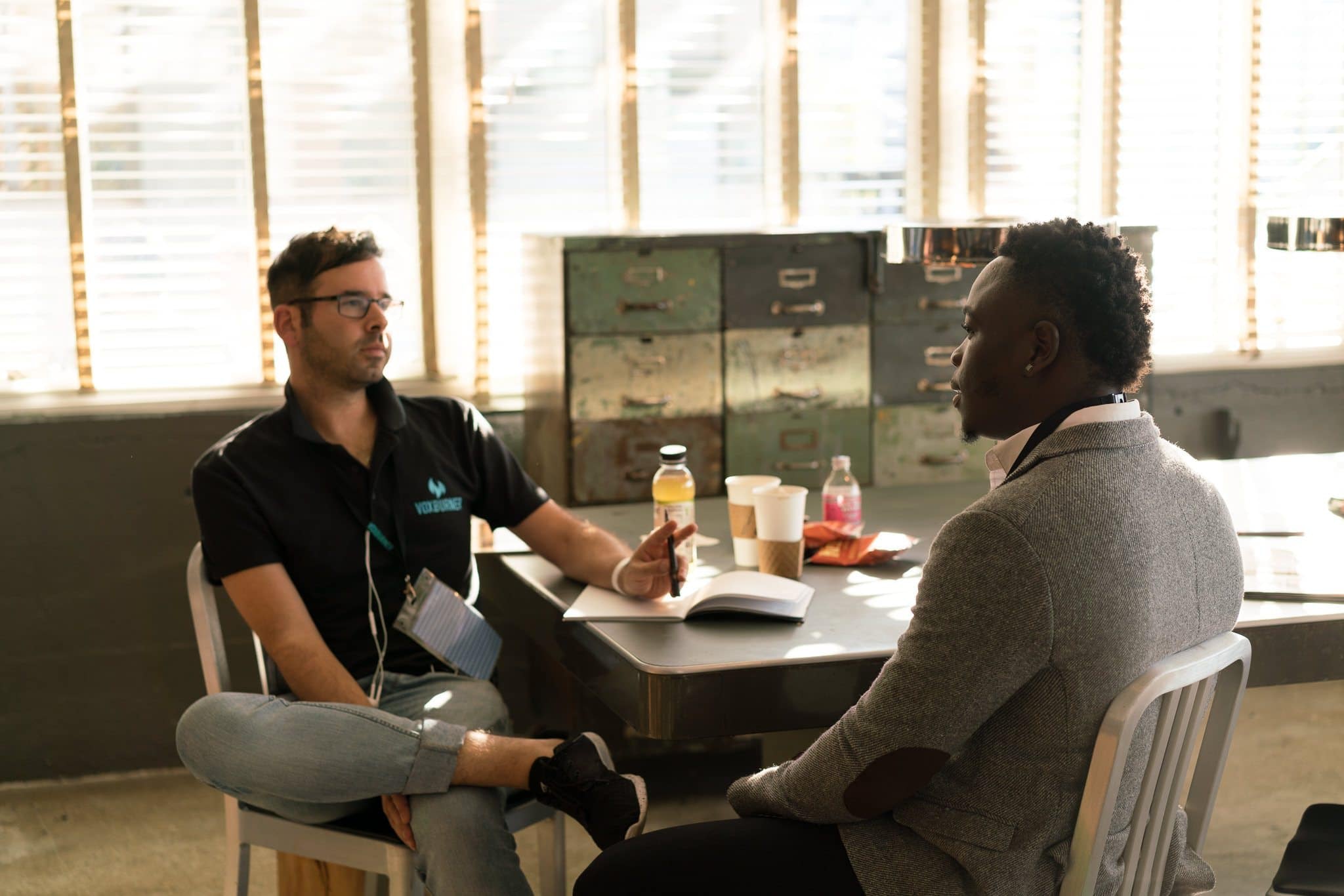
A 2018 study found that Face++ and Microsoft AI, popular facial recognition softwares that could be used to analyze candidates’ emotions for desirable traits, have been shown to assign Black males more negative emotions than their white counterparts.
Photo: Pexels
The New York City Council voted 38-4 on November 10, 2021 to pass a bill that would require hiring vendors to conduct annual bias audits of artificial intelligence (AI) use in the city’s processes and tools. Companies using AI-generated resources will be responsible for disclosing to job applicants how the technology was used in the hiring process, and must allow candidates options for alternative approaches such as having a person process their application instead. For the first time, a city the size of New York will impose fines for undisclosed or biased AI use, charging up to $1,500 per violation on employers and vendors. Lapsing into law without outgoing Mayor DeBlasio’s signature, the legislation is now set to take effect in 2023. It is a telling move in how government has started to crack down on AI use in hiring processes and foreshadows what other cities may do to combat AI-generated bias and discrimination.
AI Use in Hiring
In recent years, companies have accelerated AI deployment in their hiring processes. As the economy recovers from the devastating impacts of COVID-19 and the ensuing “Great Resignation,” emerging technologies like AI have helped companies streamline mass hiring, while reducing some operational costs. But in the rush to deploy new technological tools, hiring professionals have not adequately addressed the intended and unintended consequences of increased AI usage, including the systematized biases that machine learning algorithms may perpetuate in employment screening and hiring practices.
In 2018, Amazon found that their AI hiring software downgraded resumes that included the word “women” and those of candidates from all-women’s colleges because the company to that point did not have much of a history of hiring female engineers and computer scientists. A 2018 study found that Face++ and Microsoft AI, popular facial recognition softwares that could be used to analyze candidates’ emotions for desirable traits, have been shown to assign Black males more negative emotions than their white counterparts. Left unchecked, these biases in automated systems result in the unjustified foreclosure of opportunities for candidates from historically disadvantaged groups.
With the help of academics, industry leaders, and civil society organizations, New York City’s leadership is pressing forward with legislation in this area that will help identify and mitigate potential drawbacks in AI use. This bill could be an important step in combatting AI biases in hiring, but experts have also been wary of its various shortcomings. Groups like the Center for Democracy and Technology (CDT) have expressed concerns over the design and execution of discrimination audits based solely on race and gender, and not other variables like disability, age, and other factors. CDT also argues that the law only applies to the hiring process, leaving room for the undisclosed use of AI when determining compensation, scheduling, working conditions, and promotions.
Even if an algorithm could be less averse to demographic groups, the multiplicity of variables collected and the use of masked proxies like zip codes will still allow said algorithm to draw conclusions around their race and other protected categories with great precision
The Use of Audits
CDT also voiced concerns regarding the bill’s lack of detail on how bias audits should be carried out. As defined in the legislation, the bias audit is “an impartial evaluation by an independent auditor . . . [which tests the] automated employment decision tool to assess the tool’s disparate impact.” New York University’s Julia Stoyanovich flagged that these requirements will be “easy to meet;” vendors will be given wide latitude for interpretation, which may subsequently dilute the lines of enforceable violations.
On this point, Deb Raji, a fellow at the Mozilla Foundation and the Algorithmic Justice League and a UC Berkeley Ph.D. student, has argued that yearly audits should not be a one-off process for which vendors bear sole responsibility. Instead, she proposes that more infrastructure could guide a more-accountable audit system. That would include an audit oversight board that could help vet and support accredited third-party actors and a national reporting system that would flag instances of discrimination and potential violations. Brookings scholar Alex Engler has also surfaced similar considerations around audit integrity of employment algorithms, arguing that the data and documentation collected by the auditors should also be reviewed for their possible biases. Such claims are pertinent to the New York City law that tethers enforcement to identifiable algorithmic harms in employment applications.
Adding to the complexity of enforcement are the data AI hiring systems are trained on. Despite efforts by federal entities like the Equal Opportunity Employment Commission (EEOC) to identify and mitigate in-person biases and discrimination in the workplace, they continue. Thus, even if an algorithm could be less averse to demographic groups, the multiplicity of variables collected and the use of masked proxies like zip codes will still allow said algorithm to draw conclusions around their race and other protected categories with great precision. For example, while the Amazon hiring algorithm was not programmed to intentionally pass over female job applicants, applicants’ college choices and past experiences were enough to indicate that they were non-male, dissimilar from previous employees, and were thereby downgraded.
Best practices in employment have worked hard to obscure traits that may bias an employer in the hiring process, including a prospective employee’s race, religion, disability status, and gender identity. The use of blind interviews, especially in pre-screening, is one example of strategies to bring fairness to the process. But through the use of AI in the hiring process, these gains can be reversed by an employer’s access to and review of publicly available photos, affinity groups, and hyper-text causally associated with applicants.
More States and Municipalities Are Following Suit
Beyond New York City, other states and municipalities have taken actions to curb AI use during the hiring process. In 2019, Illinois passed the Artificial Intelligence Video Interview Act (HB 2557), which requires employers to disclose when AI is used in a video interview and allows applicants the option to have their data deleted. Following that, Maryland passed HB 1202, which prohibits the use of facial recognition during pre-employment interviews until the employer received the consent of the applicant. California’s pending bill, SB 1241, the Talent Equity for Competitive Hiring (TECH) Act, is similar to the New York City bill and requires AI used in hiring to be pre-tested for bias on a yearly basis. Earlier this month, the Attorney General for the District of Columbia sent similar draft legislation to the city council, which would hold businesses accountable for the use of biased AI algorithms in education, employment, finance, and more through mandatory audits.
While Title VII of the Civil Rights Act of 1964 explicitly prohibits employment discrimination based on race, color, religion, sex, national origin, and others, there is much to be done to enforce the law. In December 2020, ten U.S. senators, including Sen. Michael Bennet (D-Colo.), Cory Booker (D-N.J.), and Sherrod Brown (D-Ohio), issued a letter to EEOC Chair Janet Dhillon urging the Commission to investigate bias in AI-driven hiring technologies. In response, the EEOC announced in October 2021 that it is launching an initiative to examine AI biases in hiring and ensure that these tools comply with anti-discrimination and civil rights laws.
While the New York City law appears to be a first step, many potential consequences of the use of AI throughout both the hiring and employment process remain unaddressed. Policymakers interested in building off the work of NYC should ensure that subsequent audit legislation thoroughly examines biases in AI outcomes and explores the potential to automatically trigger third-party audits when disparate treatment is thought to have occurred.
This piece originally appeared in the Brookings Institution blog.