How AI Plays Into the Future of Risk Management
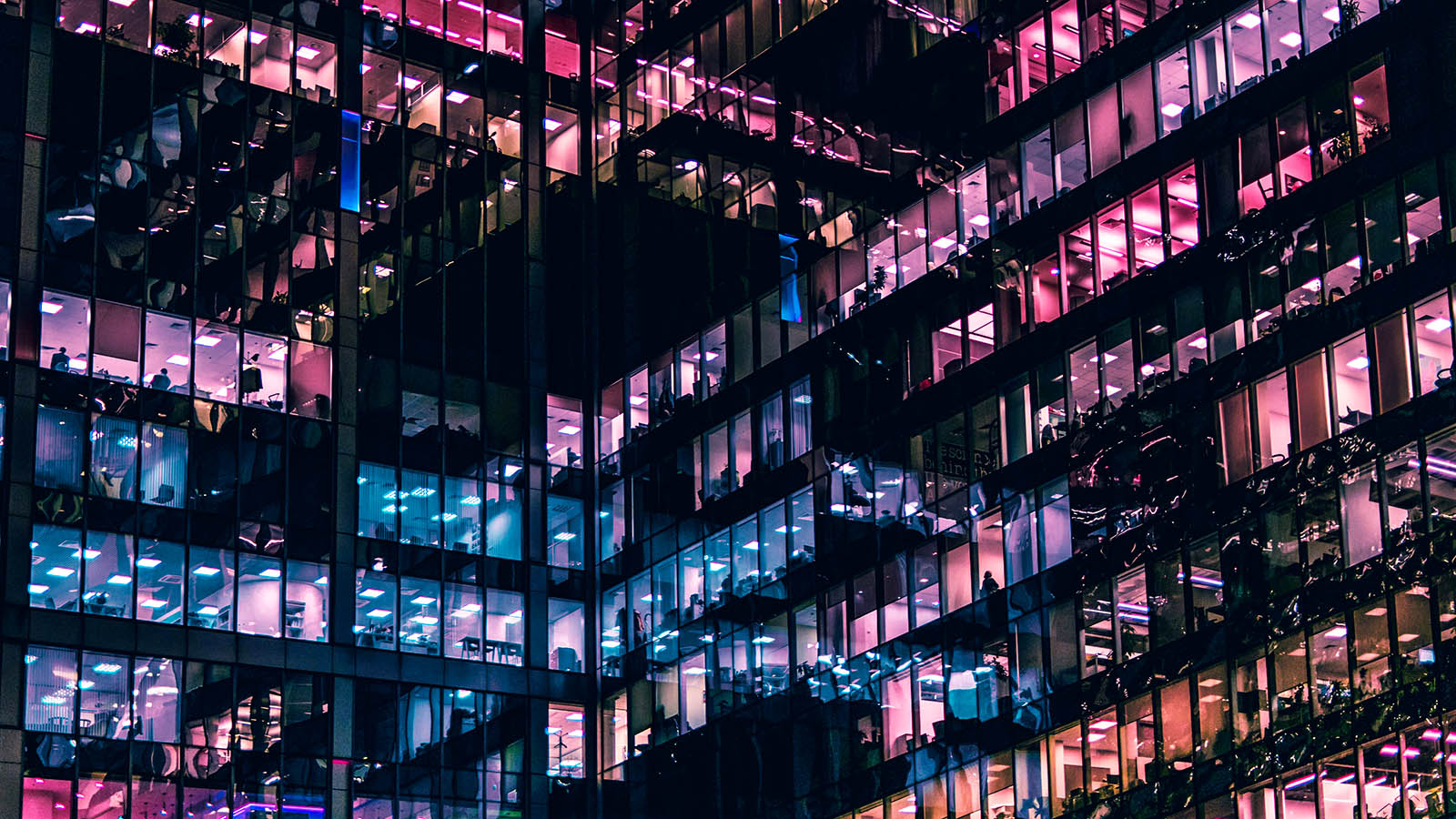
Risk analysts have been able to make sense of complex yet structured data. Although nothing in these methodologies is broken per se, the way we use data is transforming irreversibly.
Photo: Unsplash
Risk analysts have always relied on data to guide decisions toward strong growth potential and away from high-risk strategies. This used to be a fairly linear process, but now that up to 90% of our data is unstructured, information is not only difficult to organize into digestible formats but also produced in volumes that go beyond the capabilities of human analysts aided by conventional data systems.
Artificial intelligence and predictive analytics hold the promise of tackling the data burden and keeping risk predictions agile to external trends — but they need to be applied strategically to present real value and minimize business risk.
The Risk of Siloed Approaches
Historically, risk analysts have been able to make sense of complex yet structured data. Although nothing in these methodologies are broken per se, the way we use data is transforming irreversibly.
For a business discipline that should offer exactly the opposite, it’s clear that the standard practice needs to be adjusted to keep predictive analytics accurate. With the majority of all business services and consumer activity now taking place digitally, data is produced in vast, unprecedented volumes that are virtually impossible to neatly organize into structured, linear data sets for interrogation.
This is especially true for real-time data, such as payment transactions that take place minute-to-minute, or conversational data taking place across social or customer service platforms. This creates a difficult conundrum as — without both real-time and historical data insights in the hands of analysts — a vital piece of the puzzle is missing.
But it’s not just the nature of unstructured data itself that demands this change; the shockwave effects of the COVID-19 pandemic put the need for resilient risk management into sharp perspective.
The Snowball Effect
While consumer trends and economic uncertainty may have taken years to develop in the past, microtrends with the power to snowball into disruptive forces can now take place in a matter of months.
With cognitive analytics, unstructured data can not only be processed but analyzed in real-time using sub-specialisms like Natural Language Processing (NPL).
With powerful predictive analytics to hand, boardrooms stand a better chance of staying abreast of these hurdles and adjusting business models accordingly. Are consumers starting to boycott a certain manufacturer? Is a trading route no longer reliable due to political instability? Are clients indicating their desire to see cryptocurrency payment options with their service providers?
AI and predictive analytics can help to mitigate the risks businesses in every sector will face — but they will need to respond to this intelligence early.
The answers to these types of questions can help companies minimize risk, take rapid action on the future of the business and bank on decisions that build towards stability.
Setting the Right Parameters
AI and predictive analytics are powerful tools to have in the arsenal of any risk management strategy — but the ability to garner meaningful insights hinges on setting the right parameters.
You can train an algorithm to recognize phrases in conversation or one that learns how to predict consumers’ health care decisions based on their behavior patterns; the results can be predicted accurately when inherent bias has been considered upfront and accounted for in the algorithm.
The same gains are possible with risk management, but data scientists need to have an extremely clear understanding of business goals to put the right training parameters in place.
Here, business intelligence (BI) units must work alongside data scientists to guide progress. What datasets should be analyzed? Which competitors are the biggest threats? What questions or phrases should be tracked online? What constitutes a positive or negative sentiment about the brand in question?
Things get even more interesting when cognitive AI is applied on unstructured data and given the freedom to self-train, but systems should be constantly tweaked and refined to yield better results. After all, the power of this tool will only be as strong as the domain knowledge the experts from their industries can offer.
AI Risk Management in Action
The finance sector has already made strides with AI’s risk management capabilities. It’s being used to develop more accurate consumer credit scores that go deeper than just spending habits, automate the analysis of detailed legal contracts to spot anomalies and get better at spotting fraudulent behavior.
Although, risk management strategies don’t have to relate to just fraud or crime. For instance, in the data-intensive insurance sector, AI-based risk analysis helps actuaries understand the financial uncertainty and price the product for profitability while minimizing the losses.
Another example could be applied in the exploding media industry where large scale M&A are taking place, the risk of losing streaming subscribers and the need for subscriber churn prediction can affect the valuation of such acquisitions.
AI and predictive analytics can help to mitigate the risks businesses in every sector will face. By responding to this intelligence early, leaders can make active efforts to stay ahead.